AI science is experiencing a major change: there will be no single superintelligence
Team Tilde November 6, 2024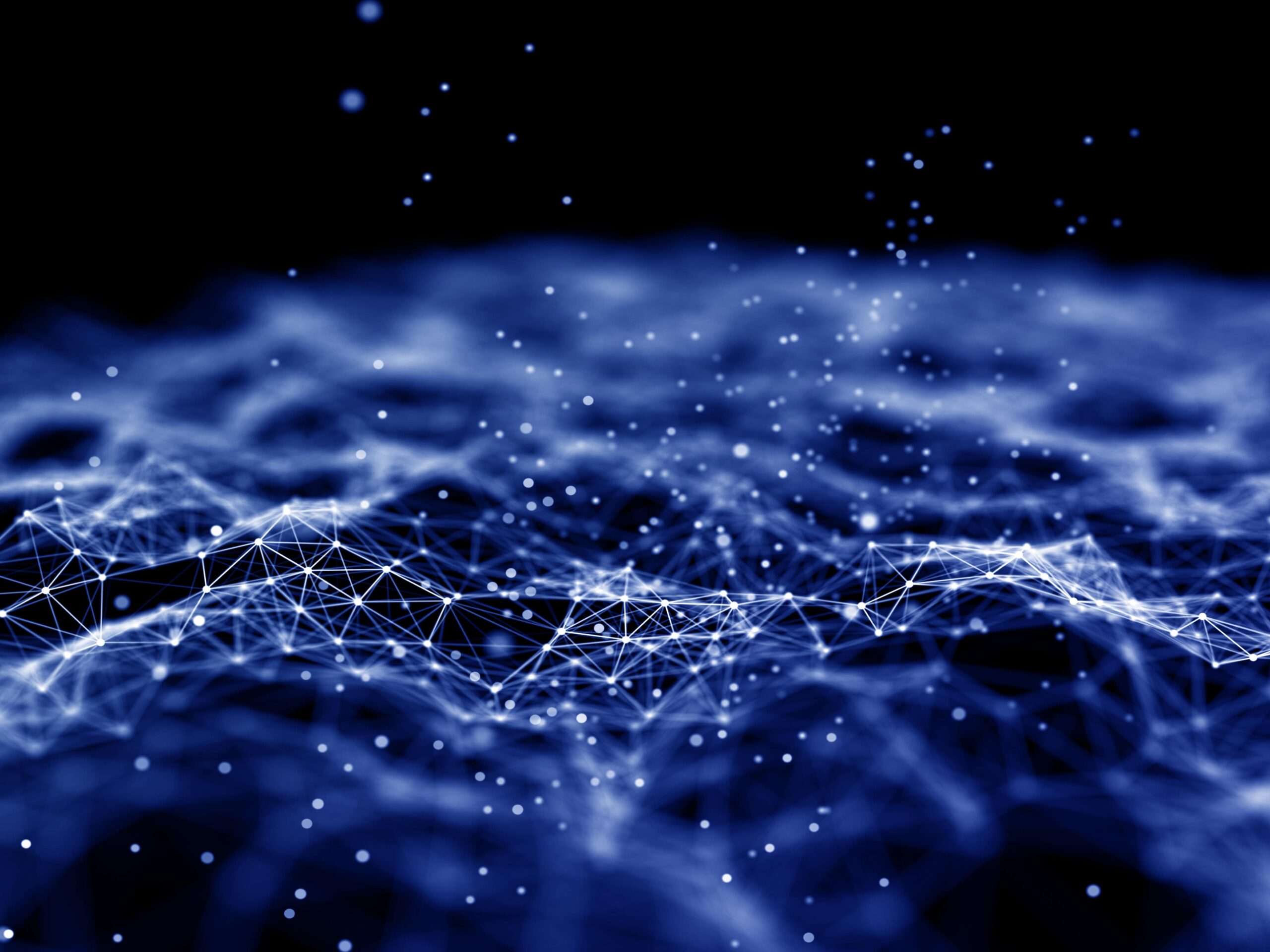
There used to be a strong belief and assumption that we need to build one superintelligence, one giant Large Language Model (LLM) capable of answering any question and resolving any problem. However, the scientific community is shifting away from that belief, moving toward an agent-based approach with specialised AI agents, where multiple smaller models are created, each excelling in its specific field. Why is this shift happening, and what will this new approach look like?
The main reason for moving towards agent-based architecture is the idea of having several smaller collaborating models-agents, i.e., experts in their own fields who achieve the best results by complementing, discussing, and amending each other. Technology developed following a human analogy usually proves itself. We can’t have one human intelligence that knows absolutely everything, so perhaps we don’t need one artificial superintelligence that knows the theory of relativity, Finnish literature, or brain surgery. Smaller specialised models-agents are likely to have fewer so-called hallucinations, i.e., fewer errors, which also occur because different areas overlap in a single model. Moreover, organisations even do not need an omniscient AI: they can choose and improve only those agents that fit their line of business.
Secondly, the use of LLMs does not follow the principles of the Green Deal. Every time an LLM answers a question, it must run through cumbersome architecture, which requires lots of calculations and entails huge energy costs. In 2017, we saw the emergence of the transformative model architecture, which is used today in all LLMs and consists of blocks with the same information-transforming formulas differing only in their coefficients (parameters). Blocks can be easily connected resulting in the large architecture of the language model: today’s language models have hundreds of billions of parameters. The training and use of such LLMs entail particularly high energy costs.
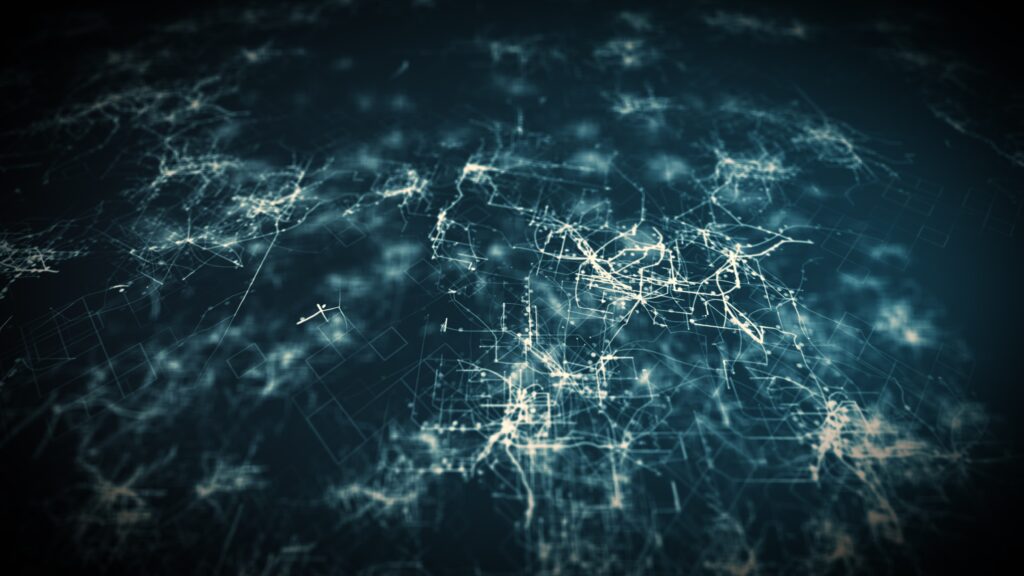
The third reason concerns data security. LLMs are simply too voluminous to easily integrate and develop in the organisation’s infrastructure. The alternative is to pass all the data to companies that manage and store these models on their servers (e.g., GPT models on OpenAI servers). Some organisations are restricted from such practices by data protection regulations, while others avoid doing so because transferring sensitive data to third parties poses additional risks. Specialised models-agents (at least those responsible for processing sensitive data) could be stored on the organisation’s servers, eliminating data protection risks.
There is another reason: the larger the language model, the more difficult is to control it. Data protection laws already allow, for example, an author of a book to require the developer to remove the book’s information from the LLM. This is challenging because it is not clear which parameters have to be adjusted and how. There are different ways of “forgetting”: e.g., the model is overwhelmed with new training data, expecting the book information to gradually fade away, or filters are added to hold back the information from the book. Unfortunately, nothing works effectively: the only proper way is to retrain the model from scratch with training data that no longer contains the author’s book. The costs are immense. Retraining smaller models-agents would be easier.
There is a major shift in the scientific community regarding the development of generative AI and LLMs, likely leading to more accurate and energy-efficient language models, thus opening the door to more extensive use.
Tilde is currently developing a general-purpose foundational multilingual LLM, TildeLM. Drawing on the scientific community’s ideas around specialized model-agents, TildeLM could later serve as the basis for smaller, distilled agents tailored to specific needs.
Comment prepared by Tilde Senior Language Technology Researcher and Vytautas Magnus University Prof. Jurgita Kapočiūtė-Dzikienė.
Transforming AI for a multilingual Europe with TildeLM
Join the movement driving digital equity and creating powerful tools for underrepresented languages.