By Dr. Toms Bergmanis, AI Researcher at Tilde
Building AI systems capable of understanding and generating human language requires vast amounts of language data. This data is the foundation for an LLM’s ability to comprehend and produce human-like language. However, the cliche that not all data is created equal stands true here. So, this distinction can make or break a model’s performance.
Judging from the model cards of the recent European and American LLMs, a vast amount of text is available for training LLMs. Yet, in reality, only some of it is high-quality, well-curated data, while the bulk is obtained opportunistically by scraping the Web.
As we at Tilde develop TildeLM, a multilingual foundational LLM, the well-known mantra “garbage in, garbage out” poses a practical problem. On the one hand, we estimate that to train a 30 billion parameter foundational LLM, we need a data set that’s around 600–700 billion words large. On the other hand, as soon as we start to investigate the quality of the available data, what seemed like a treasure trove moments ago looks more like Pandora’s box.
Pitfalls of current datasets
The most available data for training LLMs comes from two main sources: Common Crawl and Internet Archive, two repositories made by scraping millions of web pages. While these sources provide a wealth of material, they also have significant shortcomings – especially for languages other than English.
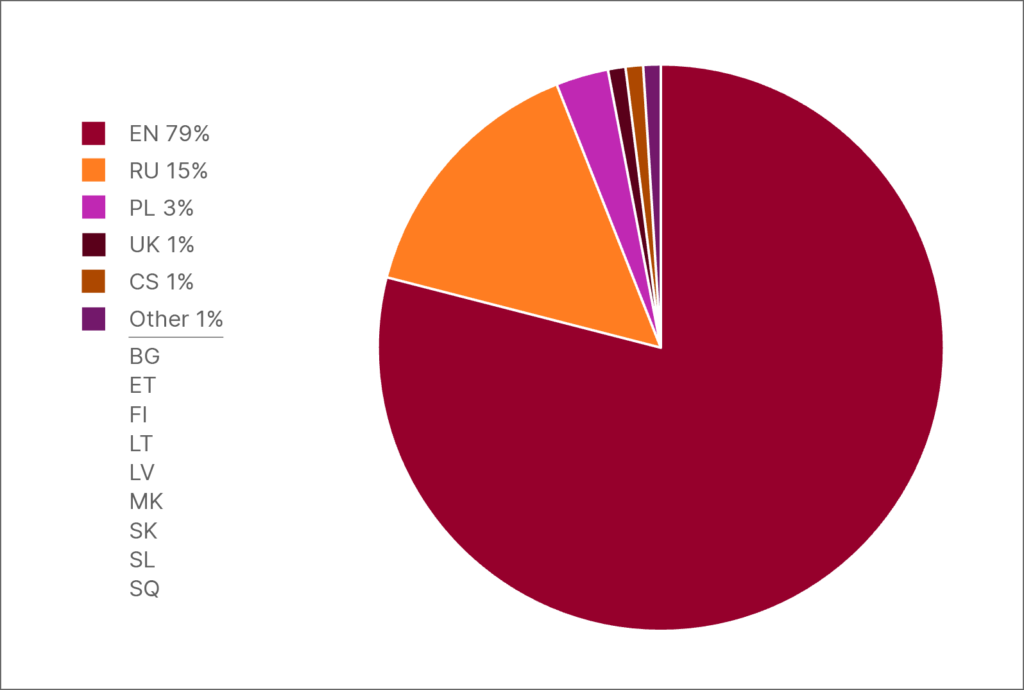
Data distribution in HPLT-v2: English vs 13 languages spoken by more than 250 million people
Regardless of the dataset, English is the most prevalent language. The number of English words in all datasets not only dwarfs any individual language but also exceeds languages from entire regions. This is the prime reason why, at present, over 90% of training data for most LLMs is in English, leaving many languages underrepresented. This imbalance perpetuates “English-centrism”, where AI models become adept at English but struggle with the nuances and cultural complexities of other languages. For speakers of underrepresented languages, this translates to lower-quality AI tools and limited access to advanced technologies.
Besides the meagre numbers, we find that a significant portion of non-English text is low-quality machine-translated content. For example, examining the 300 most frequent top-level web domains of the HPLT-v2 Latvian portion of data, we found that a staggering 25% are large machine-translated web pages.
This is a problem not only because of the poor quality of the translations but also because translations, in general, are rarely considered a good representation of a language, as they usually mimic the source language too closely. The effect is called translationese and leads to unnatural phrasing, grammatical errors, and lost cultural context. As a result, AI models trained on such data may not perform effectively in understanding or generating nuanced content in these languages.
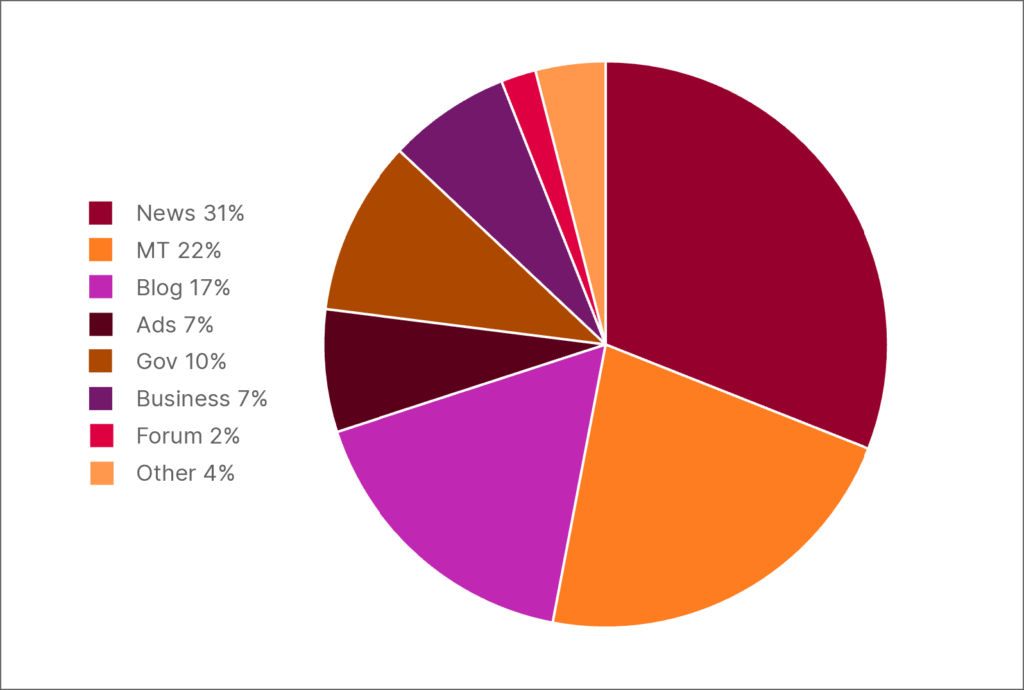
Domain label distribution among the 300 most frequent top-level web domains of the HPLT-v2 Latvian portion of data
Challenges of bias and misinformation
In addition to the problems with surface characteristics, such as incorrect grammar or noncompliance with linguistic norms, we found many more concerning problems. Examining the data from seemingly valid sources, we found content that was either inappropriate or irrelevant to our training goals. As a result, we frequently had to make judgment calls about what content was or was not acceptable. It is easy to spot materials such as pornography and justify their exclusion: porn simply fails to contribute meaningfully to language understanding. Other content, however, is much more difficult to identify, yet models trained on such content raise ethical and safety concerns.
Noteworthy is the politically charged content, especially from pro-Russian media sources. This content often carries strong anti-Western and anti-LGBT narratives, pro-Russian sentiment, and anti-Ukrainian propaganda. Many such sites are banned in the EU thanks to the efforts of organisations like the National Electronic Mass Media Council of Latvia, which also published a list of banned sites, making their exclusion easy. A lot of pro-Russian Serbian media posed a bigger challenge. While none of it is banned in the EU, we found many of the sites published hearsay or ‘expert opinions’ accompanied by fake photos to spread the Kremlin’s narratives about Western military aggression.
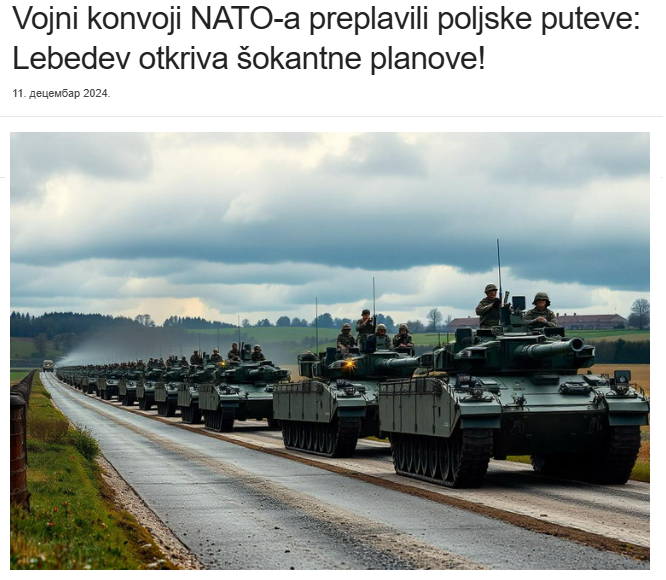
Serbian pro-Russian media site using fake images to spread fake news. Title: “NATO military convoys flooded Polish roads: Lebedev reveals shocking plans!”
Such material is problematic not only due to its political bias but also because it presents falsehoods as facts, particularly in areas like history, medicine, and social issues, to spread discord among Europeans. Training an LLM on such data risks reinforcing harmful stereotypes and misinformation, which could compromise the model’s objectivity and utility in real-world applications.
Need for quality data
It is clear that creating an effective LLM requires more than massive amounts of text; it demands high-quality, diverse, and reliable datasets. For training LLMs, we need data that provides a signal for models to learn cultural context and complex reasoning. As previous examples have illustrated, it is naive to simply rely on heaps of Web data.
Unfortunately, many high-quality datasets in languages other than English are small or fragmented.
The nature of LLM training requires long passages of text to help models grasp narrative flow and context. Without these, the LLM’s language “understanding” remains superficial. No matter how well-written, short snippets do not provide the depth needed to train a refined model.
Licensing restrictions further complicate the issue.
Yet, we found that many academic projects funded by national governments have resulted in sentence-level corpora that are useless for LLM training. Furthermore, most of such resources are intended for purely academic research and are inaccessible for commercial use, leaving commercial researchers to rely on opportunistically sourced data.
The use of low-quality data, however, doesn’t just affect LLM’s performance. It can also impact us and the languages themselves. As AI-generated content becomes more common – in emails, articles, and marketing materials – the way language is used and perceived changes. Given the fact that these tools are good at picking up and replicating linguistic detail, the widespread use of AI-generated text normalises errors or unnatural patterns over time, potentially eroding the richness of the language.
European collaboration towards better AI
Despite these challenges, there are encouraging examples of collaboration with data donors who are helping to address these issues by providing high-quality, curated datasets. Our first partner, the Estonian Language Institute (EKI), has taken proactive steps to ensure that the Estonian language is well-represented in AI training. By reaching out to offer its resources, EKI has contributed diverse materials, including literary works and government publications. These datasets are invaluable for training models to understand both formal and informal language, enabling tools that serve the community with greater accuracy and cultural sensitivity.
Similarly, SpeakLeash, a grassroots organisation in Poland, is making significant strides in preserving Polish. Run by volunteers, SpeakLeash builds and catalogues datasets specifically designed to support language in AI tools.
Both organisations have made valuable contributions to TildeLM, helping to ensure Baltic and Eastern European languages are equally represented with depth and nuance. EKI and SpeakLeash are not the only organisations that have come forward to help us. Others were the National Library of Finland, Ľ. Štúr Institute of Linguistics of the Slovak Academy of Sciences, the Slovenian Language Model initiative, the Faculty of Humanities and Social Sciences, the University of Zagreb and Univerzita Komenského v Bratislave.
Such initiatives demonstrate how local communities and organisations can actively ensure that their linguistic heritage is preserved in digital form.
What the future holds
The challenges we’ve encountered while developing TildeLM – from mere quality issues to the modern-day plague of fake news and propaganda – underscore that the “more is better” approach is fundamentally flawed. It is impossible to cross-check trillions of words in large datasets, and the challenge grows more daunting by the day. Besides, the path forward for LLMs clearly does not lie in amassing ever-larger quantities of data, as we are expected to run out of available high-quality data in the next couple of years.
Only time will tell if the future of AI lies in thoughtful collaboration between technology companies, academic institutions, and cultural organisations. The encouraging partnerships with organisations like the Estonian Language Institute and SpeakLeash demonstrate that such cooperation is achievable in principle. Now, we need to see if it can scale beyond individual success stories and result in better-quality models trained on smaller, more reliable datasets. The answer to this question may well determine whether AI can truly serve all languages and cultures equally.